limit of (2) state of Markov chain, probability of being in state $A$ or $B$ in the long termSteady State...
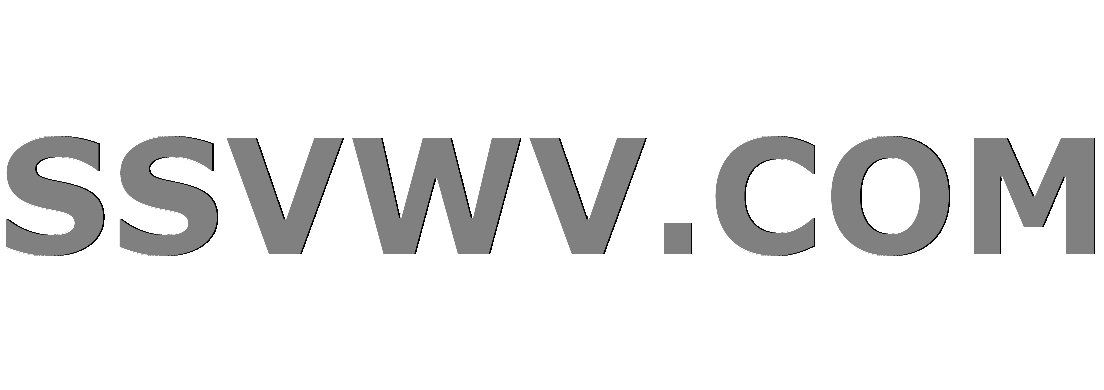
Multi tool use
How does Ehrenfest's theorem apply to the quantum harmonic oscillator?
Gaining more land
Are small insurances worth it?
What will happen if my luggage gets delayed?
Is it a Cyclops number? "Nobody" knows!
Vocabulary for giving just numbers, not a full answer
Getting the || sign while using Kurier
Why is there an extra space when I type "ls" in the Desktop directory?
What do you call someone who likes to pick fights?
After `ssh` without `-X` to a machine, is it possible to change `$DISPLAY` to make it work like `ssh -X`?
Was it really inappropriate to write a pull request for the company I interviewed with?
Would an aboleth's Phantasmal Force lair action be affected by Counterspell, Dispel Magic, and/or Slow?
When a wind turbine does not produce enough electricity how does the power company compensate for the loss?
(Codewars) Linked Lists - Remove Duplicates
Is it possible to avoid unpacking when merging Association?
Can't make sense of a paragraph from Lovecraft
Why couldn't the separatists legally leave the Republic?
Giving a career talk in my old university, how prominently should I tell students my salary?
Does Christianity allow for believing on someone else's behalf?
Why does cron require MTA for logging?
Is this Paypal Github SDK reference really a dangerous site?
What would be the most expensive material to an intergalactic society?
The meaning of ‘otherwise’
Expressing logarithmic equations without logs
limit of (2) state of Markov chain, probability of being in state $A$ or $B$ in the long term
Steady State Markov ChainMarkov chain probability that a state changesLong term probability in Markov Chainsmarkov chain: 2 state chainFind steady state of continuous time Markov chainExpected payoff of a 2-State Markov ChainUnderstanding the “first step analysis” of absorbing Markov chainsA question on Random Walks and Markov ChainProbability $mathbb{P}[X_N = 1 | X_0 = 1]$ for a Markov Chain over $mathbb{X} = {1,2,3}$How to find probability transition matrix for continuous time markov chain?
$begingroup$
Let there be a frog jumping on two spots $A$ and $B$ such that
$$mathbb P[X_n=Bmid X_{n-1}=A]=alpha=:p_{AB}\
mathbb P[X_n=Amid X_{n-1}=B]=beta=:p_{BA}$$
and so $p_{AA}=1-alpha, p_{BB}=1-beta$, where $X_n$ is the position of the frog at time $ninBbb N$
The transition matrix is $P=begin{pmatrix}1-alpha & alpha\beta & 1-beta end{pmatrix}$
I found the eigenvalues $1$ and $1-alpha-beta$ and diagonalized the matrix to get the transition matrix for $n$ steps $P^n=begin{pmatrix}frac{beta}{alpha+beta}+frac{alpha}{alpha+beta}(1-alpha-beta)^n & frac{alpha}{alpha+beta}-frac{alpha}{alpha+beta}(1-alpha-beta)^n\
frac{beta}{alpha+beta}+frac{beta}{alpha+beta}(1-alpha-beta)^n & frac{alpha}{alpha+beta}-frac{beta}{alpha+beta}(1-alpha-beta)^n
end{pmatrix}$ which clearly converges to
$$P^infty:=begin{pmatrix}frac{beta}{alpha+beta} & frac{alpha}{alpha+beta}\
frac{beta}{alpha+beta} & frac{alpha}{alpha+beta}end{pmatrix}$$ as long as $0<alpha+beta<2$
How do you understand what $P^infty$ is? Can we say that after an eternity the frog is on spot $A$ with probability $frac{beta}{alpha+beta}$ and on spot $B$ with probability $frac{alpha}{alpha+beta}$? Why?
stochastic-processes markov-chains conditional-probability
$endgroup$
add a comment |
$begingroup$
Let there be a frog jumping on two spots $A$ and $B$ such that
$$mathbb P[X_n=Bmid X_{n-1}=A]=alpha=:p_{AB}\
mathbb P[X_n=Amid X_{n-1}=B]=beta=:p_{BA}$$
and so $p_{AA}=1-alpha, p_{BB}=1-beta$, where $X_n$ is the position of the frog at time $ninBbb N$
The transition matrix is $P=begin{pmatrix}1-alpha & alpha\beta & 1-beta end{pmatrix}$
I found the eigenvalues $1$ and $1-alpha-beta$ and diagonalized the matrix to get the transition matrix for $n$ steps $P^n=begin{pmatrix}frac{beta}{alpha+beta}+frac{alpha}{alpha+beta}(1-alpha-beta)^n & frac{alpha}{alpha+beta}-frac{alpha}{alpha+beta}(1-alpha-beta)^n\
frac{beta}{alpha+beta}+frac{beta}{alpha+beta}(1-alpha-beta)^n & frac{alpha}{alpha+beta}-frac{beta}{alpha+beta}(1-alpha-beta)^n
end{pmatrix}$ which clearly converges to
$$P^infty:=begin{pmatrix}frac{beta}{alpha+beta} & frac{alpha}{alpha+beta}\
frac{beta}{alpha+beta} & frac{alpha}{alpha+beta}end{pmatrix}$$ as long as $0<alpha+beta<2$
How do you understand what $P^infty$ is? Can we say that after an eternity the frog is on spot $A$ with probability $frac{beta}{alpha+beta}$ and on spot $B$ with probability $frac{alpha}{alpha+beta}$? Why?
stochastic-processes markov-chains conditional-probability
$endgroup$
$begingroup$
Let for a state $i$, let $V_i(n)=sum_{k=0}^{n-1} mathsf 1_{{X_k=i}}$. The ergodic theorem states that $$mathbb P(lim_{ntoinfty} V_i(n)/n = pi_i)=1, $$ where $pi$ is the (unique) stationary distribution of the Markov chain. In other words, the fraction of time spent in state $i$ converges to $pi_i$ almost surely.
$endgroup$
– Math1000
yesterday
add a comment |
$begingroup$
Let there be a frog jumping on two spots $A$ and $B$ such that
$$mathbb P[X_n=Bmid X_{n-1}=A]=alpha=:p_{AB}\
mathbb P[X_n=Amid X_{n-1}=B]=beta=:p_{BA}$$
and so $p_{AA}=1-alpha, p_{BB}=1-beta$, where $X_n$ is the position of the frog at time $ninBbb N$
The transition matrix is $P=begin{pmatrix}1-alpha & alpha\beta & 1-beta end{pmatrix}$
I found the eigenvalues $1$ and $1-alpha-beta$ and diagonalized the matrix to get the transition matrix for $n$ steps $P^n=begin{pmatrix}frac{beta}{alpha+beta}+frac{alpha}{alpha+beta}(1-alpha-beta)^n & frac{alpha}{alpha+beta}-frac{alpha}{alpha+beta}(1-alpha-beta)^n\
frac{beta}{alpha+beta}+frac{beta}{alpha+beta}(1-alpha-beta)^n & frac{alpha}{alpha+beta}-frac{beta}{alpha+beta}(1-alpha-beta)^n
end{pmatrix}$ which clearly converges to
$$P^infty:=begin{pmatrix}frac{beta}{alpha+beta} & frac{alpha}{alpha+beta}\
frac{beta}{alpha+beta} & frac{alpha}{alpha+beta}end{pmatrix}$$ as long as $0<alpha+beta<2$
How do you understand what $P^infty$ is? Can we say that after an eternity the frog is on spot $A$ with probability $frac{beta}{alpha+beta}$ and on spot $B$ with probability $frac{alpha}{alpha+beta}$? Why?
stochastic-processes markov-chains conditional-probability
$endgroup$
Let there be a frog jumping on two spots $A$ and $B$ such that
$$mathbb P[X_n=Bmid X_{n-1}=A]=alpha=:p_{AB}\
mathbb P[X_n=Amid X_{n-1}=B]=beta=:p_{BA}$$
and so $p_{AA}=1-alpha, p_{BB}=1-beta$, where $X_n$ is the position of the frog at time $ninBbb N$
The transition matrix is $P=begin{pmatrix}1-alpha & alpha\beta & 1-beta end{pmatrix}$
I found the eigenvalues $1$ and $1-alpha-beta$ and diagonalized the matrix to get the transition matrix for $n$ steps $P^n=begin{pmatrix}frac{beta}{alpha+beta}+frac{alpha}{alpha+beta}(1-alpha-beta)^n & frac{alpha}{alpha+beta}-frac{alpha}{alpha+beta}(1-alpha-beta)^n\
frac{beta}{alpha+beta}+frac{beta}{alpha+beta}(1-alpha-beta)^n & frac{alpha}{alpha+beta}-frac{beta}{alpha+beta}(1-alpha-beta)^n
end{pmatrix}$ which clearly converges to
$$P^infty:=begin{pmatrix}frac{beta}{alpha+beta} & frac{alpha}{alpha+beta}\
frac{beta}{alpha+beta} & frac{alpha}{alpha+beta}end{pmatrix}$$ as long as $0<alpha+beta<2$
How do you understand what $P^infty$ is? Can we say that after an eternity the frog is on spot $A$ with probability $frac{beta}{alpha+beta}$ and on spot $B$ with probability $frac{alpha}{alpha+beta}$? Why?
stochastic-processes markov-chains conditional-probability
stochastic-processes markov-chains conditional-probability
edited 2 days ago
John Cataldo
asked 2 days ago


John CataldoJohn Cataldo
1,1881316
1,1881316
$begingroup$
Let for a state $i$, let $V_i(n)=sum_{k=0}^{n-1} mathsf 1_{{X_k=i}}$. The ergodic theorem states that $$mathbb P(lim_{ntoinfty} V_i(n)/n = pi_i)=1, $$ where $pi$ is the (unique) stationary distribution of the Markov chain. In other words, the fraction of time spent in state $i$ converges to $pi_i$ almost surely.
$endgroup$
– Math1000
yesterday
add a comment |
$begingroup$
Let for a state $i$, let $V_i(n)=sum_{k=0}^{n-1} mathsf 1_{{X_k=i}}$. The ergodic theorem states that $$mathbb P(lim_{ntoinfty} V_i(n)/n = pi_i)=1, $$ where $pi$ is the (unique) stationary distribution of the Markov chain. In other words, the fraction of time spent in state $i$ converges to $pi_i$ almost surely.
$endgroup$
– Math1000
yesterday
$begingroup$
Let for a state $i$, let $V_i(n)=sum_{k=0}^{n-1} mathsf 1_{{X_k=i}}$. The ergodic theorem states that $$mathbb P(lim_{ntoinfty} V_i(n)/n = pi_i)=1, $$ where $pi$ is the (unique) stationary distribution of the Markov chain. In other words, the fraction of time spent in state $i$ converges to $pi_i$ almost surely.
$endgroup$
– Math1000
yesterday
$begingroup$
Let for a state $i$, let $V_i(n)=sum_{k=0}^{n-1} mathsf 1_{{X_k=i}}$. The ergodic theorem states that $$mathbb P(lim_{ntoinfty} V_i(n)/n = pi_i)=1, $$ where $pi$ is the (unique) stationary distribution of the Markov chain. In other words, the fraction of time spent in state $i$ converges to $pi_i$ almost surely.
$endgroup$
– Math1000
yesterday
add a comment |
0
active
oldest
votes
Your Answer
StackExchange.ifUsing("editor", function () {
return StackExchange.using("mathjaxEditing", function () {
StackExchange.MarkdownEditor.creationCallbacks.add(function (editor, postfix) {
StackExchange.mathjaxEditing.prepareWmdForMathJax(editor, postfix, [["$", "$"], ["\\(","\\)"]]);
});
});
}, "mathjax-editing");
StackExchange.ready(function() {
var channelOptions = {
tags: "".split(" "),
id: "69"
};
initTagRenderer("".split(" "), "".split(" "), channelOptions);
StackExchange.using("externalEditor", function() {
// Have to fire editor after snippets, if snippets enabled
if (StackExchange.settings.snippets.snippetsEnabled) {
StackExchange.using("snippets", function() {
createEditor();
});
}
else {
createEditor();
}
});
function createEditor() {
StackExchange.prepareEditor({
heartbeatType: 'answer',
autoActivateHeartbeat: false,
convertImagesToLinks: true,
noModals: true,
showLowRepImageUploadWarning: true,
reputationToPostImages: 10,
bindNavPrevention: true,
postfix: "",
imageUploader: {
brandingHtml: "Powered by u003ca class="icon-imgur-white" href="https://imgur.com/"u003eu003c/au003e",
contentPolicyHtml: "User contributions licensed under u003ca href="https://creativecommons.org/licenses/by-sa/3.0/"u003ecc by-sa 3.0 with attribution requiredu003c/au003e u003ca href="https://stackoverflow.com/legal/content-policy"u003e(content policy)u003c/au003e",
allowUrls: true
},
noCode: true, onDemand: true,
discardSelector: ".discard-answer"
,immediatelyShowMarkdownHelp:true
});
}
});
Sign up or log in
StackExchange.ready(function () {
StackExchange.helpers.onClickDraftSave('#login-link');
});
Sign up using Google
Sign up using Facebook
Sign up using Email and Password
Post as a guest
Required, but never shown
StackExchange.ready(
function () {
StackExchange.openid.initPostLogin('.new-post-login', 'https%3a%2f%2fmath.stackexchange.com%2fquestions%2f3140304%2flimit-of-2-state-of-markov-chain-probability-of-being-in-state-a-or-b-in%23new-answer', 'question_page');
}
);
Post as a guest
Required, but never shown
0
active
oldest
votes
0
active
oldest
votes
active
oldest
votes
active
oldest
votes
Thanks for contributing an answer to Mathematics Stack Exchange!
- Please be sure to answer the question. Provide details and share your research!
But avoid …
- Asking for help, clarification, or responding to other answers.
- Making statements based on opinion; back them up with references or personal experience.
Use MathJax to format equations. MathJax reference.
To learn more, see our tips on writing great answers.
Sign up or log in
StackExchange.ready(function () {
StackExchange.helpers.onClickDraftSave('#login-link');
});
Sign up using Google
Sign up using Facebook
Sign up using Email and Password
Post as a guest
Required, but never shown
StackExchange.ready(
function () {
StackExchange.openid.initPostLogin('.new-post-login', 'https%3a%2f%2fmath.stackexchange.com%2fquestions%2f3140304%2flimit-of-2-state-of-markov-chain-probability-of-being-in-state-a-or-b-in%23new-answer', 'question_page');
}
);
Post as a guest
Required, but never shown
Sign up or log in
StackExchange.ready(function () {
StackExchange.helpers.onClickDraftSave('#login-link');
});
Sign up using Google
Sign up using Facebook
Sign up using Email and Password
Post as a guest
Required, but never shown
Sign up or log in
StackExchange.ready(function () {
StackExchange.helpers.onClickDraftSave('#login-link');
});
Sign up using Google
Sign up using Facebook
Sign up using Email and Password
Post as a guest
Required, but never shown
Sign up or log in
StackExchange.ready(function () {
StackExchange.helpers.onClickDraftSave('#login-link');
});
Sign up using Google
Sign up using Facebook
Sign up using Email and Password
Sign up using Google
Sign up using Facebook
Sign up using Email and Password
Post as a guest
Required, but never shown
Required, but never shown
Required, but never shown
Required, but never shown
Required, but never shown
Required, but never shown
Required, but never shown
Required, but never shown
Required, but never shown
7Hj,GIGTvANOBRRyNg,ODfJO6JNf5diVIoTErmj7L9B77Posrti9rJ 26QIj0rPioP o vxJtCoj6SNpw1yqDCo6kKH,t
$begingroup$
Let for a state $i$, let $V_i(n)=sum_{k=0}^{n-1} mathsf 1_{{X_k=i}}$. The ergodic theorem states that $$mathbb P(lim_{ntoinfty} V_i(n)/n = pi_i)=1, $$ where $pi$ is the (unique) stationary distribution of the Markov chain. In other words, the fraction of time spent in state $i$ converges to $pi_i$ almost surely.
$endgroup$
– Math1000
yesterday