non homogeneous exponential distributionExponential Distribution with changing (time-varying) rate...
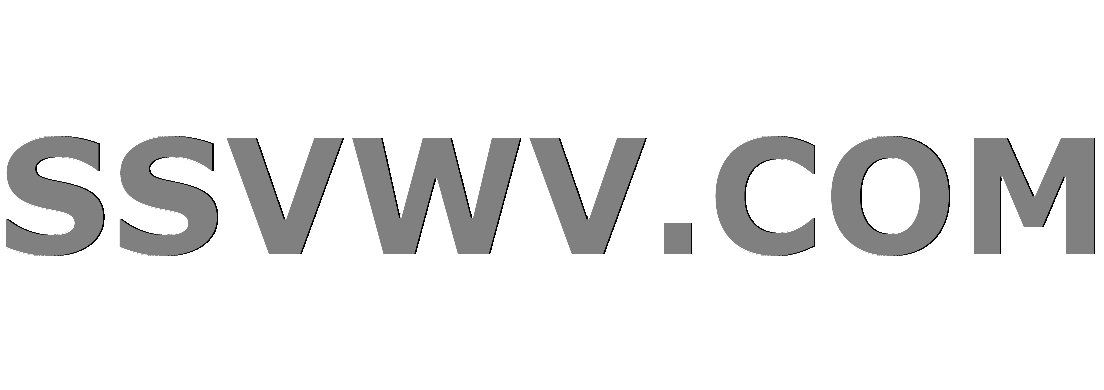
Multi tool use
Western buddy movie with a supernatural twist where a woman turns into an eagle at the end
How could indestructible materials be used in power generation?
Infinite Abelian subgroup of infinite non Abelian group example
How can saying a song's name be a copyright violation?
Emailing HOD to enhance faculty application
Watching something be written to a file live with tail
If human space travel is limited by the G force vulnerability, is there a way to counter G forces?
Stopping power of mountain vs road bike
Reserved de-dupe rules
A reference to a well-known characterization of scattered compact spaces
Intersection of two sorted vectors in C++
Is it possible to run Internet Explorer on OS X El Capitan?
Why is the 'in' operator throwing an error with a string literal instead of logging false?
What is the most common color to indicate the input-field is disabled?
Why is it a bad idea to hire a hitman to eliminate most corrupt politicians?
How do I write bicross product symbols in latex?
Did Shadowfax go to Valinor?
1960's book about a plague that kills all white people
How do conventional missiles fly?
Were any external disk drives stacked vertically?
How to draw the figure with four pentagons?
How to take photos in burst mode, without vibration?
Is "remove commented out code" correct English?
How can I make my BBEG immortal short of making them a Lich or Vampire?
non homogeneous exponential distribution
Exponential Distribution with changing (time-varying) rate parameterReversing Exponential Distributions (kind of)Probability distribution of number of waiting customers in front of a counterBirth-Death process with shifted exponential distributionDetermine lambda parameter of exponential distribution from covarianceLink between Poisson and Exponential distributionWhat do we mean by rate in the exponential distribution?Exponential distribution stochasticExpected Value of Poisson Distribution depending on Random Variable with Exponential DistributionDoes the truncated exponential distribution preserves the memoryless property?
$begingroup$
Given an exponential waiting time with rate $lambda$ we know that the distribution for the waiting time would be
$$ f(T=t) = lambda e^{-lambda t} $$
now, if we assume that the rate is not constant, let´s say $lambda(t)$, I am wondering if the distribution would be just
$$ f(T=t) = lambda(t) e^{-int_0^t lambda(t) dt} $$
or not. And if not, what would be the distribution? I cannot find any references for this distribution, so any help is appreciated.
probability exponential-distribution
$endgroup$
add a comment |
$begingroup$
Given an exponential waiting time with rate $lambda$ we know that the distribution for the waiting time would be
$$ f(T=t) = lambda e^{-lambda t} $$
now, if we assume that the rate is not constant, let´s say $lambda(t)$, I am wondering if the distribution would be just
$$ f(T=t) = lambda(t) e^{-int_0^t lambda(t) dt} $$
or not. And if not, what would be the distribution? I cannot find any references for this distribution, so any help is appreciated.
probability exponential-distribution
$endgroup$
1
$begingroup$
Please note that actually, $P(T=t)=0$ for every $t$.
$endgroup$
– Did
Dec 19 '17 at 20:52
add a comment |
$begingroup$
Given an exponential waiting time with rate $lambda$ we know that the distribution for the waiting time would be
$$ f(T=t) = lambda e^{-lambda t} $$
now, if we assume that the rate is not constant, let´s say $lambda(t)$, I am wondering if the distribution would be just
$$ f(T=t) = lambda(t) e^{-int_0^t lambda(t) dt} $$
or not. And if not, what would be the distribution? I cannot find any references for this distribution, so any help is appreciated.
probability exponential-distribution
$endgroup$
Given an exponential waiting time with rate $lambda$ we know that the distribution for the waiting time would be
$$ f(T=t) = lambda e^{-lambda t} $$
now, if we assume that the rate is not constant, let´s say $lambda(t)$, I am wondering if the distribution would be just
$$ f(T=t) = lambda(t) e^{-int_0^t lambda(t) dt} $$
or not. And if not, what would be the distribution? I cannot find any references for this distribution, so any help is appreciated.
probability exponential-distribution
probability exponential-distribution
edited Jul 20 '18 at 11:56
Francisco
asked Dec 19 '17 at 15:29
FranciscoFrancisco
320110
320110
1
$begingroup$
Please note that actually, $P(T=t)=0$ for every $t$.
$endgroup$
– Did
Dec 19 '17 at 20:52
add a comment |
1
$begingroup$
Please note that actually, $P(T=t)=0$ for every $t$.
$endgroup$
– Did
Dec 19 '17 at 20:52
1
1
$begingroup$
Please note that actually, $P(T=t)=0$ for every $t$.
$endgroup$
– Did
Dec 19 '17 at 20:52
$begingroup$
Please note that actually, $P(T=t)=0$ for every $t$.
$endgroup$
– Did
Dec 19 '17 at 20:52
add a comment |
3 Answers
3
active
oldest
votes
$begingroup$
In actuarial science, the function $lambda(t)$ - more commonly notated as $mu_t$ - is known as the force of mortality or the hazard function. When the force of mortality is not constant, you obtain distributions that are not exponential. For life-insurance context, you may want to look up the Gompertz distribution and Makeham distribution.
Given a force of mortality $mu_t$ for random variable $X$, the relationship between its CDF $F_X$, its PDF $f_X$, and $mu_t$ is
$$mu_t=dfrac{f_X(t)}{1-F_X(t)}=-dfrac{S^{prime}_X(t)}{S_X(t)}=-dfrac{text{d}}{text{d}t}[ln S_X(t)]$$
where $S_X = 1 - F_X$ is commonly called the "survival function." From this, you can integrate to obtain a formula for $S_X$. Given the property that $F_X(0)=0$ (do you see why?), we can obtain $S_X(0) = 1$, hence
$$int_{0}^{t}mu_stext{ d}s=-int_{0}^{t}dfrac{text{d}}{text{d}t}[ln S_X(t)]text{ d}t=-[ln S_X(t)-ln 1]=-ln S_X(t)$$
from which we obtain
$$S_X(t)=expleft[-int_{0}^{t}mu_stext{ d}s right]$$
and thus
$$F_X(t)=1-expleft[-int_{0}^{t}mu_stext{ d}s right]$$
and you can differentiate to obtain
$$f_X(t)=mu_texpleft[-int_{0}^{t}mu_stext{ d}s right]$$
matching your form above.
$endgroup$
add a comment |
$begingroup$
Except for your error about the meaning of a density, you are correct.
The easy way to figure this out is to consider the discrete version. Suppose the probability of an event happening in $[t,t+dt]$ is $lambda(t) dt$. Then the probability of waiting for at least n intervals with no event is
$$P=prod_{i=1}^n (1-dt lambda((i-1)dt).$$
Now set $dt=t/n$ and send $n to infty$. To compute the limit, compute the exponential of its logarithm. The logarithm is
$$log(P)=sum_{i=1}^n log left ( 1-frac{t}{n} lambda left ( (i-1)frac{t}{n} right ) right ).$$
By linear approximation
$$log(P)=o(1)+sum_{i=1}^n -frac{t}{n} lambda left ( (i-1) frac{t}{n} right ).$$
The sum is a Riemann sum, and the higher order correction vanishes in the $n to infty$ limit, so you get the limit of $log(P)$ as an integral. The quantity you just calculated is then $P(T>t)$.
By the way, the main place you would find this is in references to continuous time, discrete space, time-inhomogeneous Markov chains, where it appears as the holding time distribution.
$endgroup$
add a comment |
$begingroup$
To sample a value from a non-homogeneous exponential distribution you can follow this steps
S1. Sample $x$ from homogeneous exponential with rate 1
S2. Calculate $Lambda^{-1} (x)$
where $Lambda(t)$ is the intensity function (the integral of the rate).
The random variable $Lambda^{-1} (x)$ has a non-homogeneous distribution with rate $lambda(t)$.
A reference could be the paper "Generating Nonhomogeneous Poisson Processes" by Raghu Pasupathy.
$endgroup$
add a comment |
Your Answer
StackExchange.ifUsing("editor", function () {
return StackExchange.using("mathjaxEditing", function () {
StackExchange.MarkdownEditor.creationCallbacks.add(function (editor, postfix) {
StackExchange.mathjaxEditing.prepareWmdForMathJax(editor, postfix, [["$", "$"], ["\\(","\\)"]]);
});
});
}, "mathjax-editing");
StackExchange.ready(function() {
var channelOptions = {
tags: "".split(" "),
id: "69"
};
initTagRenderer("".split(" "), "".split(" "), channelOptions);
StackExchange.using("externalEditor", function() {
// Have to fire editor after snippets, if snippets enabled
if (StackExchange.settings.snippets.snippetsEnabled) {
StackExchange.using("snippets", function() {
createEditor();
});
}
else {
createEditor();
}
});
function createEditor() {
StackExchange.prepareEditor({
heartbeatType: 'answer',
autoActivateHeartbeat: false,
convertImagesToLinks: true,
noModals: true,
showLowRepImageUploadWarning: true,
reputationToPostImages: 10,
bindNavPrevention: true,
postfix: "",
imageUploader: {
brandingHtml: "Powered by u003ca class="icon-imgur-white" href="https://imgur.com/"u003eu003c/au003e",
contentPolicyHtml: "User contributions licensed under u003ca href="https://creativecommons.org/licenses/by-sa/3.0/"u003ecc by-sa 3.0 with attribution requiredu003c/au003e u003ca href="https://stackoverflow.com/legal/content-policy"u003e(content policy)u003c/au003e",
allowUrls: true
},
noCode: true, onDemand: true,
discardSelector: ".discard-answer"
,immediatelyShowMarkdownHelp:true
});
}
});
Sign up or log in
StackExchange.ready(function () {
StackExchange.helpers.onClickDraftSave('#login-link');
});
Sign up using Google
Sign up using Facebook
Sign up using Email and Password
Post as a guest
Required, but never shown
StackExchange.ready(
function () {
StackExchange.openid.initPostLogin('.new-post-login', 'https%3a%2f%2fmath.stackexchange.com%2fquestions%2f2573284%2fnon-homogeneous-exponential-distribution%23new-answer', 'question_page');
}
);
Post as a guest
Required, but never shown
3 Answers
3
active
oldest
votes
3 Answers
3
active
oldest
votes
active
oldest
votes
active
oldest
votes
$begingroup$
In actuarial science, the function $lambda(t)$ - more commonly notated as $mu_t$ - is known as the force of mortality or the hazard function. When the force of mortality is not constant, you obtain distributions that are not exponential. For life-insurance context, you may want to look up the Gompertz distribution and Makeham distribution.
Given a force of mortality $mu_t$ for random variable $X$, the relationship between its CDF $F_X$, its PDF $f_X$, and $mu_t$ is
$$mu_t=dfrac{f_X(t)}{1-F_X(t)}=-dfrac{S^{prime}_X(t)}{S_X(t)}=-dfrac{text{d}}{text{d}t}[ln S_X(t)]$$
where $S_X = 1 - F_X$ is commonly called the "survival function." From this, you can integrate to obtain a formula for $S_X$. Given the property that $F_X(0)=0$ (do you see why?), we can obtain $S_X(0) = 1$, hence
$$int_{0}^{t}mu_stext{ d}s=-int_{0}^{t}dfrac{text{d}}{text{d}t}[ln S_X(t)]text{ d}t=-[ln S_X(t)-ln 1]=-ln S_X(t)$$
from which we obtain
$$S_X(t)=expleft[-int_{0}^{t}mu_stext{ d}s right]$$
and thus
$$F_X(t)=1-expleft[-int_{0}^{t}mu_stext{ d}s right]$$
and you can differentiate to obtain
$$f_X(t)=mu_texpleft[-int_{0}^{t}mu_stext{ d}s right]$$
matching your form above.
$endgroup$
add a comment |
$begingroup$
In actuarial science, the function $lambda(t)$ - more commonly notated as $mu_t$ - is known as the force of mortality or the hazard function. When the force of mortality is not constant, you obtain distributions that are not exponential. For life-insurance context, you may want to look up the Gompertz distribution and Makeham distribution.
Given a force of mortality $mu_t$ for random variable $X$, the relationship between its CDF $F_X$, its PDF $f_X$, and $mu_t$ is
$$mu_t=dfrac{f_X(t)}{1-F_X(t)}=-dfrac{S^{prime}_X(t)}{S_X(t)}=-dfrac{text{d}}{text{d}t}[ln S_X(t)]$$
where $S_X = 1 - F_X$ is commonly called the "survival function." From this, you can integrate to obtain a formula for $S_X$. Given the property that $F_X(0)=0$ (do you see why?), we can obtain $S_X(0) = 1$, hence
$$int_{0}^{t}mu_stext{ d}s=-int_{0}^{t}dfrac{text{d}}{text{d}t}[ln S_X(t)]text{ d}t=-[ln S_X(t)-ln 1]=-ln S_X(t)$$
from which we obtain
$$S_X(t)=expleft[-int_{0}^{t}mu_stext{ d}s right]$$
and thus
$$F_X(t)=1-expleft[-int_{0}^{t}mu_stext{ d}s right]$$
and you can differentiate to obtain
$$f_X(t)=mu_texpleft[-int_{0}^{t}mu_stext{ d}s right]$$
matching your form above.
$endgroup$
add a comment |
$begingroup$
In actuarial science, the function $lambda(t)$ - more commonly notated as $mu_t$ - is known as the force of mortality or the hazard function. When the force of mortality is not constant, you obtain distributions that are not exponential. For life-insurance context, you may want to look up the Gompertz distribution and Makeham distribution.
Given a force of mortality $mu_t$ for random variable $X$, the relationship between its CDF $F_X$, its PDF $f_X$, and $mu_t$ is
$$mu_t=dfrac{f_X(t)}{1-F_X(t)}=-dfrac{S^{prime}_X(t)}{S_X(t)}=-dfrac{text{d}}{text{d}t}[ln S_X(t)]$$
where $S_X = 1 - F_X$ is commonly called the "survival function." From this, you can integrate to obtain a formula for $S_X$. Given the property that $F_X(0)=0$ (do you see why?), we can obtain $S_X(0) = 1$, hence
$$int_{0}^{t}mu_stext{ d}s=-int_{0}^{t}dfrac{text{d}}{text{d}t}[ln S_X(t)]text{ d}t=-[ln S_X(t)-ln 1]=-ln S_X(t)$$
from which we obtain
$$S_X(t)=expleft[-int_{0}^{t}mu_stext{ d}s right]$$
and thus
$$F_X(t)=1-expleft[-int_{0}^{t}mu_stext{ d}s right]$$
and you can differentiate to obtain
$$f_X(t)=mu_texpleft[-int_{0}^{t}mu_stext{ d}s right]$$
matching your form above.
$endgroup$
In actuarial science, the function $lambda(t)$ - more commonly notated as $mu_t$ - is known as the force of mortality or the hazard function. When the force of mortality is not constant, you obtain distributions that are not exponential. For life-insurance context, you may want to look up the Gompertz distribution and Makeham distribution.
Given a force of mortality $mu_t$ for random variable $X$, the relationship between its CDF $F_X$, its PDF $f_X$, and $mu_t$ is
$$mu_t=dfrac{f_X(t)}{1-F_X(t)}=-dfrac{S^{prime}_X(t)}{S_X(t)}=-dfrac{text{d}}{text{d}t}[ln S_X(t)]$$
where $S_X = 1 - F_X$ is commonly called the "survival function." From this, you can integrate to obtain a formula for $S_X$. Given the property that $F_X(0)=0$ (do you see why?), we can obtain $S_X(0) = 1$, hence
$$int_{0}^{t}mu_stext{ d}s=-int_{0}^{t}dfrac{text{d}}{text{d}t}[ln S_X(t)]text{ d}t=-[ln S_X(t)-ln 1]=-ln S_X(t)$$
from which we obtain
$$S_X(t)=expleft[-int_{0}^{t}mu_stext{ d}s right]$$
and thus
$$F_X(t)=1-expleft[-int_{0}^{t}mu_stext{ d}s right]$$
and you can differentiate to obtain
$$f_X(t)=mu_texpleft[-int_{0}^{t}mu_stext{ d}s right]$$
matching your form above.
answered Dec 19 '17 at 16:05
ClarinetistClarinetist
11.1k42878
11.1k42878
add a comment |
add a comment |
$begingroup$
Except for your error about the meaning of a density, you are correct.
The easy way to figure this out is to consider the discrete version. Suppose the probability of an event happening in $[t,t+dt]$ is $lambda(t) dt$. Then the probability of waiting for at least n intervals with no event is
$$P=prod_{i=1}^n (1-dt lambda((i-1)dt).$$
Now set $dt=t/n$ and send $n to infty$. To compute the limit, compute the exponential of its logarithm. The logarithm is
$$log(P)=sum_{i=1}^n log left ( 1-frac{t}{n} lambda left ( (i-1)frac{t}{n} right ) right ).$$
By linear approximation
$$log(P)=o(1)+sum_{i=1}^n -frac{t}{n} lambda left ( (i-1) frac{t}{n} right ).$$
The sum is a Riemann sum, and the higher order correction vanishes in the $n to infty$ limit, so you get the limit of $log(P)$ as an integral. The quantity you just calculated is then $P(T>t)$.
By the way, the main place you would find this is in references to continuous time, discrete space, time-inhomogeneous Markov chains, where it appears as the holding time distribution.
$endgroup$
add a comment |
$begingroup$
Except for your error about the meaning of a density, you are correct.
The easy way to figure this out is to consider the discrete version. Suppose the probability of an event happening in $[t,t+dt]$ is $lambda(t) dt$. Then the probability of waiting for at least n intervals with no event is
$$P=prod_{i=1}^n (1-dt lambda((i-1)dt).$$
Now set $dt=t/n$ and send $n to infty$. To compute the limit, compute the exponential of its logarithm. The logarithm is
$$log(P)=sum_{i=1}^n log left ( 1-frac{t}{n} lambda left ( (i-1)frac{t}{n} right ) right ).$$
By linear approximation
$$log(P)=o(1)+sum_{i=1}^n -frac{t}{n} lambda left ( (i-1) frac{t}{n} right ).$$
The sum is a Riemann sum, and the higher order correction vanishes in the $n to infty$ limit, so you get the limit of $log(P)$ as an integral. The quantity you just calculated is then $P(T>t)$.
By the way, the main place you would find this is in references to continuous time, discrete space, time-inhomogeneous Markov chains, where it appears as the holding time distribution.
$endgroup$
add a comment |
$begingroup$
Except for your error about the meaning of a density, you are correct.
The easy way to figure this out is to consider the discrete version. Suppose the probability of an event happening in $[t,t+dt]$ is $lambda(t) dt$. Then the probability of waiting for at least n intervals with no event is
$$P=prod_{i=1}^n (1-dt lambda((i-1)dt).$$
Now set $dt=t/n$ and send $n to infty$. To compute the limit, compute the exponential of its logarithm. The logarithm is
$$log(P)=sum_{i=1}^n log left ( 1-frac{t}{n} lambda left ( (i-1)frac{t}{n} right ) right ).$$
By linear approximation
$$log(P)=o(1)+sum_{i=1}^n -frac{t}{n} lambda left ( (i-1) frac{t}{n} right ).$$
The sum is a Riemann sum, and the higher order correction vanishes in the $n to infty$ limit, so you get the limit of $log(P)$ as an integral. The quantity you just calculated is then $P(T>t)$.
By the way, the main place you would find this is in references to continuous time, discrete space, time-inhomogeneous Markov chains, where it appears as the holding time distribution.
$endgroup$
Except for your error about the meaning of a density, you are correct.
The easy way to figure this out is to consider the discrete version. Suppose the probability of an event happening in $[t,t+dt]$ is $lambda(t) dt$. Then the probability of waiting for at least n intervals with no event is
$$P=prod_{i=1}^n (1-dt lambda((i-1)dt).$$
Now set $dt=t/n$ and send $n to infty$. To compute the limit, compute the exponential of its logarithm. The logarithm is
$$log(P)=sum_{i=1}^n log left ( 1-frac{t}{n} lambda left ( (i-1)frac{t}{n} right ) right ).$$
By linear approximation
$$log(P)=o(1)+sum_{i=1}^n -frac{t}{n} lambda left ( (i-1) frac{t}{n} right ).$$
The sum is a Riemann sum, and the higher order correction vanishes in the $n to infty$ limit, so you get the limit of $log(P)$ as an integral. The quantity you just calculated is then $P(T>t)$.
By the way, the main place you would find this is in references to continuous time, discrete space, time-inhomogeneous Markov chains, where it appears as the holding time distribution.
edited Dec 19 '17 at 18:05
answered Dec 19 '17 at 16:01
IanIan
68.9k25392
68.9k25392
add a comment |
add a comment |
$begingroup$
To sample a value from a non-homogeneous exponential distribution you can follow this steps
S1. Sample $x$ from homogeneous exponential with rate 1
S2. Calculate $Lambda^{-1} (x)$
where $Lambda(t)$ is the intensity function (the integral of the rate).
The random variable $Lambda^{-1} (x)$ has a non-homogeneous distribution with rate $lambda(t)$.
A reference could be the paper "Generating Nonhomogeneous Poisson Processes" by Raghu Pasupathy.
$endgroup$
add a comment |
$begingroup$
To sample a value from a non-homogeneous exponential distribution you can follow this steps
S1. Sample $x$ from homogeneous exponential with rate 1
S2. Calculate $Lambda^{-1} (x)$
where $Lambda(t)$ is the intensity function (the integral of the rate).
The random variable $Lambda^{-1} (x)$ has a non-homogeneous distribution with rate $lambda(t)$.
A reference could be the paper "Generating Nonhomogeneous Poisson Processes" by Raghu Pasupathy.
$endgroup$
add a comment |
$begingroup$
To sample a value from a non-homogeneous exponential distribution you can follow this steps
S1. Sample $x$ from homogeneous exponential with rate 1
S2. Calculate $Lambda^{-1} (x)$
where $Lambda(t)$ is the intensity function (the integral of the rate).
The random variable $Lambda^{-1} (x)$ has a non-homogeneous distribution with rate $lambda(t)$.
A reference could be the paper "Generating Nonhomogeneous Poisson Processes" by Raghu Pasupathy.
$endgroup$
To sample a value from a non-homogeneous exponential distribution you can follow this steps
S1. Sample $x$ from homogeneous exponential with rate 1
S2. Calculate $Lambda^{-1} (x)$
where $Lambda(t)$ is the intensity function (the integral of the rate).
The random variable $Lambda^{-1} (x)$ has a non-homogeneous distribution with rate $lambda(t)$.
A reference could be the paper "Generating Nonhomogeneous Poisson Processes" by Raghu Pasupathy.
answered Mar 19 at 0:20
FranciscoFrancisco
320110
320110
add a comment |
add a comment |
Thanks for contributing an answer to Mathematics Stack Exchange!
- Please be sure to answer the question. Provide details and share your research!
But avoid …
- Asking for help, clarification, or responding to other answers.
- Making statements based on opinion; back them up with references or personal experience.
Use MathJax to format equations. MathJax reference.
To learn more, see our tips on writing great answers.
Sign up or log in
StackExchange.ready(function () {
StackExchange.helpers.onClickDraftSave('#login-link');
});
Sign up using Google
Sign up using Facebook
Sign up using Email and Password
Post as a guest
Required, but never shown
StackExchange.ready(
function () {
StackExchange.openid.initPostLogin('.new-post-login', 'https%3a%2f%2fmath.stackexchange.com%2fquestions%2f2573284%2fnon-homogeneous-exponential-distribution%23new-answer', 'question_page');
}
);
Post as a guest
Required, but never shown
Sign up or log in
StackExchange.ready(function () {
StackExchange.helpers.onClickDraftSave('#login-link');
});
Sign up using Google
Sign up using Facebook
Sign up using Email and Password
Post as a guest
Required, but never shown
Sign up or log in
StackExchange.ready(function () {
StackExchange.helpers.onClickDraftSave('#login-link');
});
Sign up using Google
Sign up using Facebook
Sign up using Email and Password
Post as a guest
Required, but never shown
Sign up or log in
StackExchange.ready(function () {
StackExchange.helpers.onClickDraftSave('#login-link');
});
Sign up using Google
Sign up using Facebook
Sign up using Email and Password
Sign up using Google
Sign up using Facebook
Sign up using Email and Password
Post as a guest
Required, but never shown
Required, but never shown
Required, but never shown
Required, but never shown
Required, but never shown
Required, but never shown
Required, but never shown
Required, but never shown
Required, but never shown
gRCF6f,s2bWPAtZAzFx5PyQw kQjqTdefd5tbSkqyv F7Y1IHvZdrMD5cyMqnm sMHv
1
$begingroup$
Please note that actually, $P(T=t)=0$ for every $t$.
$endgroup$
– Did
Dec 19 '17 at 20:52